The Zizi Show
A Deepfake Drag Cabaret
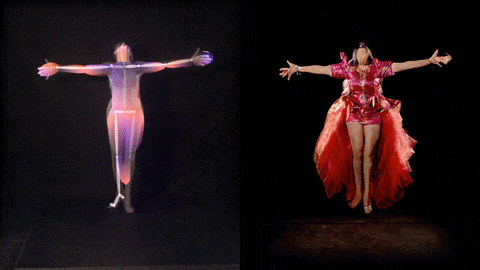
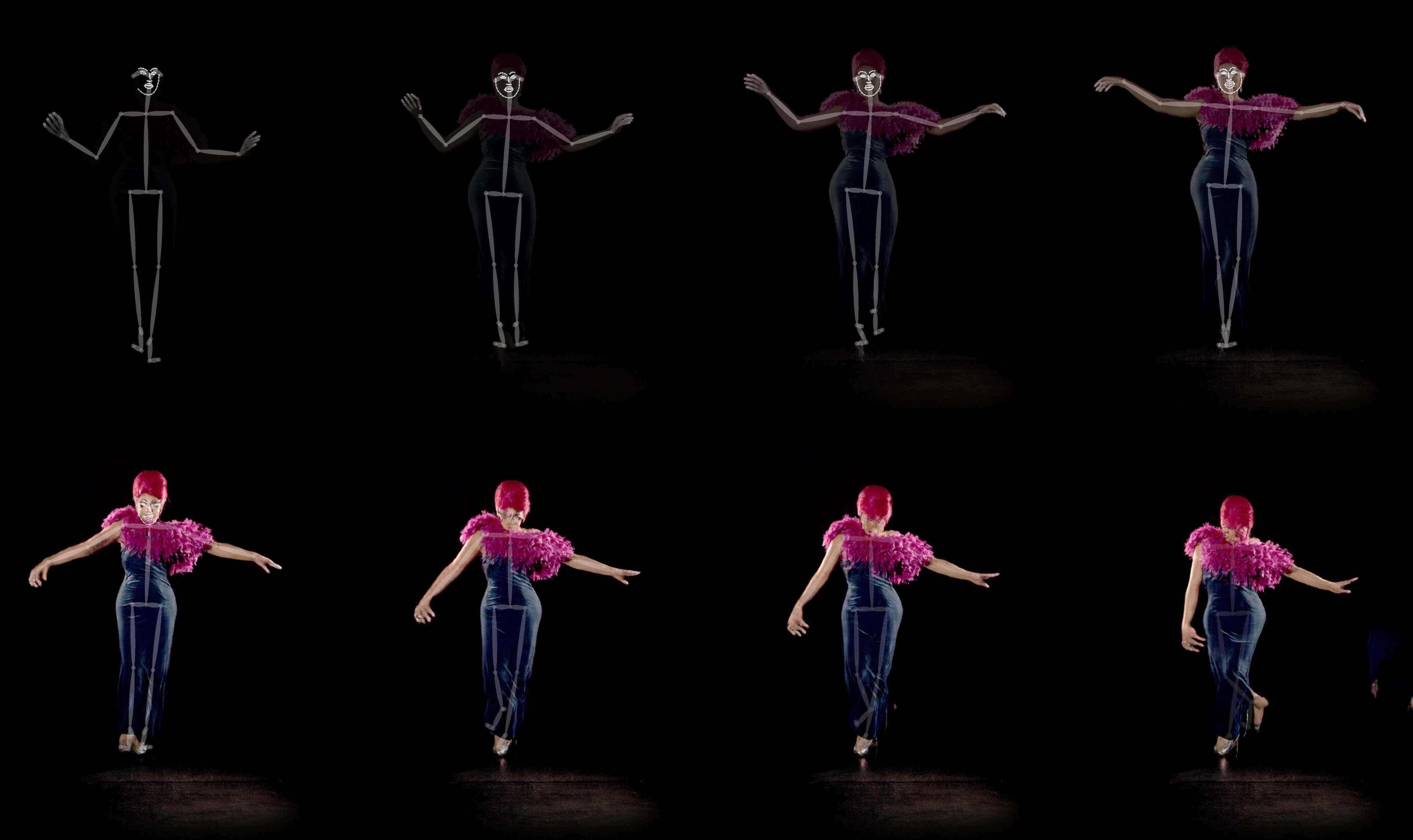
"The Zizi Project aims to bring together two things I love, artificial intelligence, and the world of drag performance. Drag has allowed me to dig into some of the social issues built into machine learning technology and explore the layers of construction and social bias in AI.
Working closely with friends from the London drag scene, in Zizi we have created a ‘deepfake’ virtual cabaret. Deepfake technology has enabled us to collaborate with machine learning to do drag, consentually re-animating each others forms. We trained our deepfakes on 21 extremely talented performers - perhaps proving that drag queens, drag kings and drag things will never be replaced by artificial intelligence.
The Zizi Project pushes the boundaries of both drag and AI to discover what AI can teach us about drag – and what drag can teach us about AI.”
- Jake Elwes
Team
Jake Elwes
Artist, Coder & Producer
Me The Drag Queen
Director of Drag
Toby Elwes
Director of Photography
Royal Vauxhall Tavern & The Apple Tree
Dataset Filming Locations / Drag Venues
Alexander Hill
Web & Development for
zizi.ai
Professor Drew Hemment
Research Director
Dr Joe Parslow
Performance / Research Consultant
The first iteration of The Zizi Show was commissioned by The New Real at University of Edinburgh and The Alan Turing Institute as an online experience for Edinburgh International Festival (2021).
Comissioned by the V&A as a multi-channel video installation in 2023 for the opening of the new Photography Center.
Special thanks to Tom Francome for the documentary film, Charlie Baker for help with sound, Luke & Anneke Elwes, Dave Cross + John & James at the RVT
Email zizidragshow@gmail.com to request access to the interactive web app zizi.ai
Cast
Music & Performances
This Is My Life, Shirley Bassey
Movement by Me The Drag
Queen
You Make Me Feel (Mighty Real), Sylvester
Movement by Chiyo
Raise Your Glass, Pink
Movement by Lilly
SnatchDragon
I Am What I Am, Douglas Hodge
Movement by Me The Drag
Queen
Freedom! '90, George Michael
Movement by Mark
Anthony
Sweet Dreams (Live), Beyoncé
Movement by Cara
Melle
Five Years, David Bowie
Movement by Ruby
Wednesday
Transition movement by Wet Mess
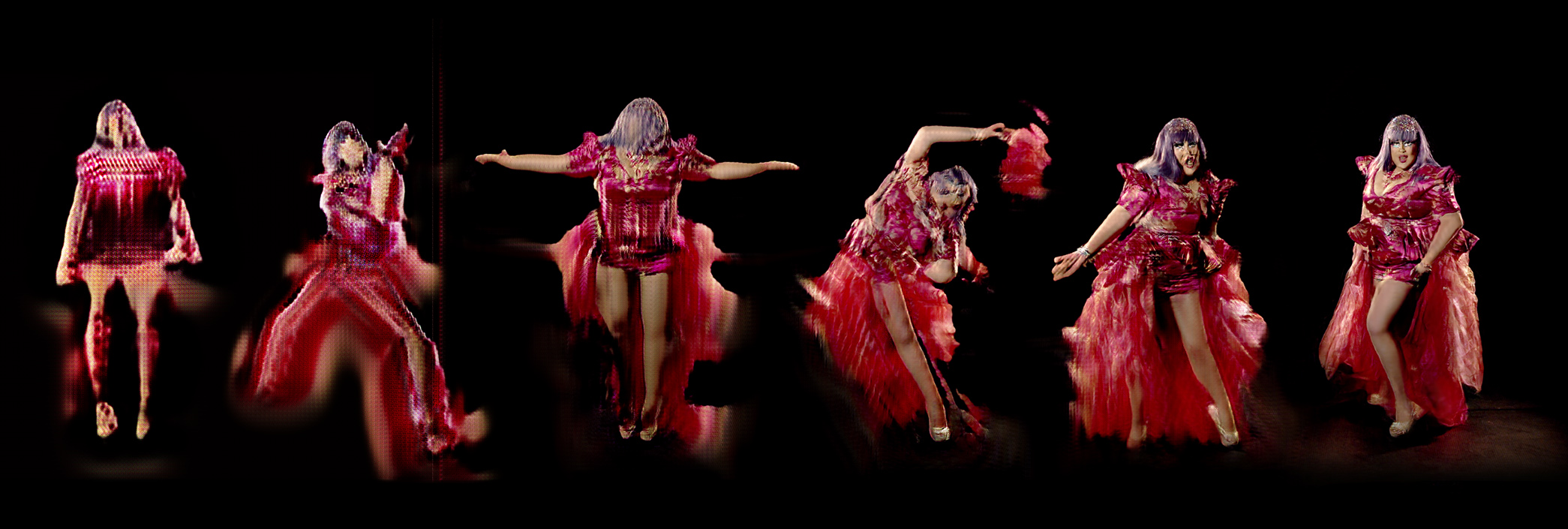
Process
Machine learning – teaching computers to learn from data – and specifically deepfake technology, has been used to construct all the videos you see. To produce a deepfake, you start by training a neural network1 on a dataset of images.
This dataset contains the original images (video frames) of the real-life person, as well as a graphic tracking the position of their skeleton, facial features, and silhouette.
Creating deepfakes begins with training a neural network to try to recreate the original image of this person based on just their skeleton tracking (illustrated below). The neural network aims to get as close as possible to the original and does this by being given an accuracy score.2 Once it has learnt to do this it can then start producing deepfakes.
Below, you can see the iterative training process of a neural network as it learns how to create images of the drag queen an burlesque artist Lilly SnatchDragon:
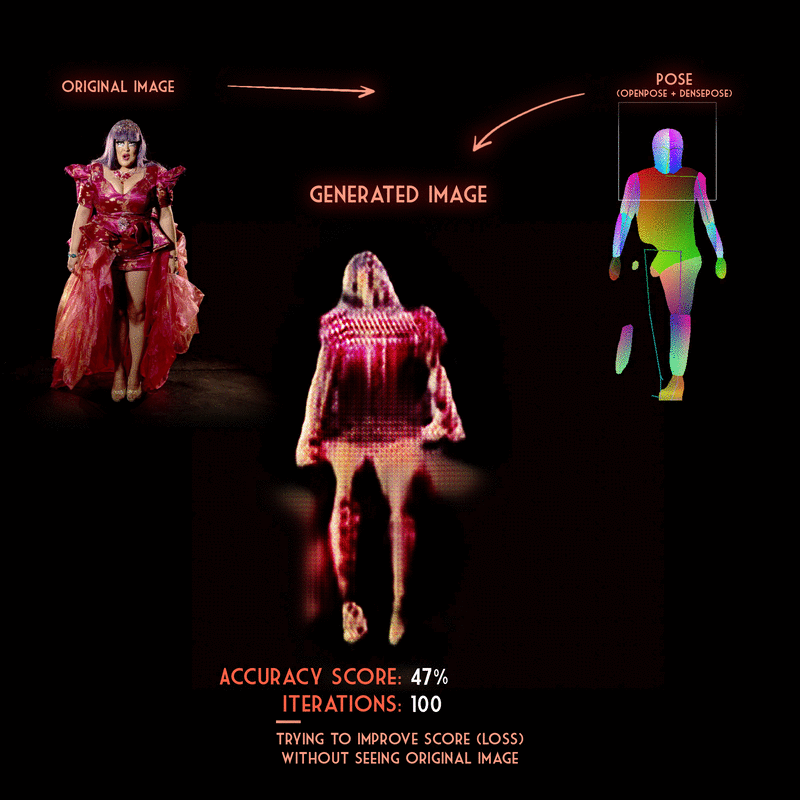
Using machine learning, this process iterates and improves until it can create new, fake faces which are indistinguishable from the real. For Zizi, the method I use is called Video-to-Video Synthesis. 3
Once the neural network has been training for, let's say, three days, it is ready to be fed new movements. Anyone can now control the deepfake body by running skeleton tracking on a new video and then feeding these into the neural network.
These visuals below show how new deepfake images of Lilly SnatchDragon can be generated from the trained neural network (here with her movement controlled by Me The Drag Queen).
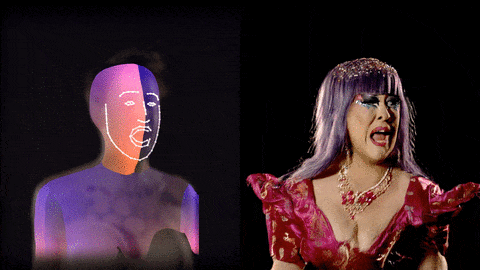
This process was repeated to create deepfakes of all 21 of our wonderful, diverse drag cast.
The ‘Zizi’ character was created by simultaneously training on images of all of the performers. Not knowing how to differentiate between the bodies, the result is an amalgamation, a ‘queering’ of the data.
Facial recognition algorithms (and deepfake technology) currently have difficulty recognising trans, queer and other marginalised identities, because they are often made by heteronormative white people.4,5
The project asks whether making deepfakes using queer identities becomes a means of assimilation or inclusivity… or more a techno-activist method of dirtying and obfuscating the systems used to collect data on us.
The Zizi project aims to critically examine these techniques using a dataset of drag performers, in the process exposing the workings of the black box which is artificial intelligence.
Footnotes
1. For more information see DeepAI.org glossary - 'neural network'^
2. This accuracy score (loss) is calculated using the gradient descent learning algorithm.^
3. Video-to-Video Synthesis is a conditional generative adversarial network (cGAN) developed by Wang et al. (Nvidia & MIT), NeurIPS, 2018. It uses OpenPose (2018) for skeleton tracking and DensePose (2018) for silhouette estimation. This technique also uses Flownet (2016) to take into account the motion in the video.^
4. Gender recognition or gender reductionism? The social implications of embedded gender recognition systems Hamidi, F., Scheuerman, M.K. and Branham, S.M. (2018).^
5. Excavating AI: The Politics of Images in Machine Learning Training Sets Kate Crawford and Trevor Paglen.^
Further reading: Algorithmic Justice League Resources, AI Artists - Ethical AI Resources, New Real Magazine